What Is AI-Ready Data? Why It Matters and How to Prepare It
Let’s say you have loads of data, not AI-ready data, with lots of messy entries like: some in all caps, some full of emojis, others with abbreviations never seen before.
This spells out inconsistency in full, because when you run such a model, the recommendations it will give: laughably off, like instead of suggesting beach resorts to someone who said “I love the sun and chilling,” it might recommend ski lodges.
Why do you think that is? Let’s go back to the drawing board, because clean and standardized data is everything, adding labels, filtering out irrelevant entries, can make sure each piece of your data has context.
Only then will the model start working the way it’s been envisioned..
This distinction shows the difference between a system that just functions and one that thrives.
Key Takeaway
- Understanding what AI-ready Data is all about.
- The importance of having clean, structured AI-ready Data.
- Actionable steps to prepare your data for AI use.
- Exploring Real-Life Examples of AI-Ready Data in Action.
What Is AI-Ready Data?
AI-ready data refers to any data that has been properly prepared and organized to optimize its usefulness for use in artificial intelligence applications. This idea goes beyond simply having a lot of data; it emphasizes the data’s quality, structure, and relevancy, making it possible for AI algorithms to process and analyze it effectively and produce insightful results.
Key characteristics of AI-ready data
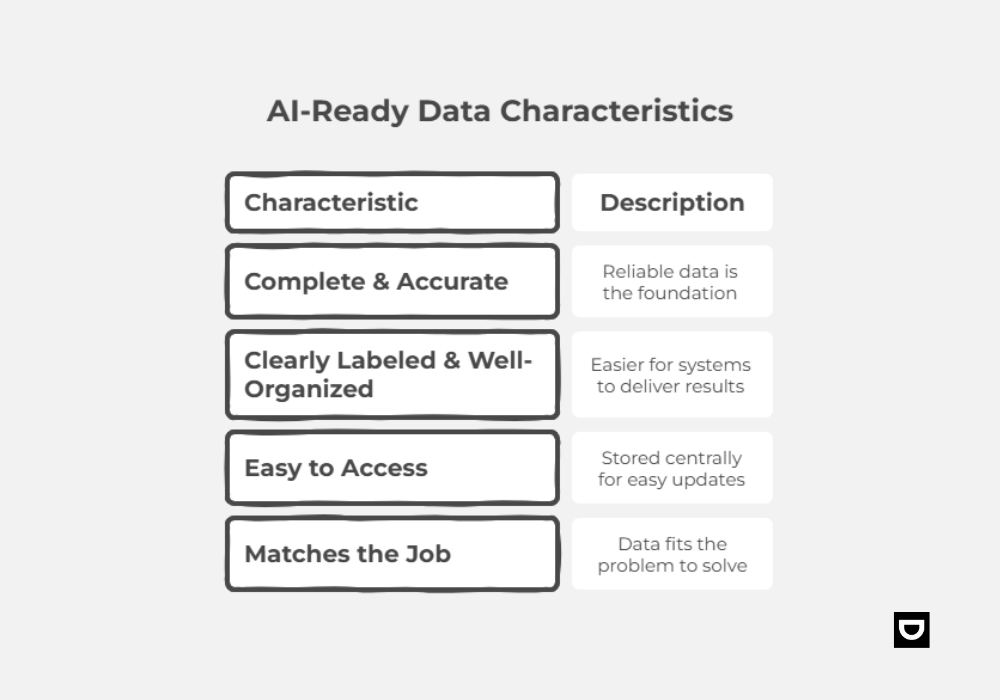
Is the Data Complete & Accurate?
Does your data meet and cover all the important details? If certain pieces are missing or the numbers don’t add up, the system can’t learn or make decisions accurately. Think of it like trying to follow a map that has missing or incomplete roads.
What does this mean? You’re bound to get lost. However, reliable data is the foundation for any tool that tries to spot patterns or make predictions.
Is it Clearly Labeled and Well-Organized?
Picture a spreadsheet where the columns keep changing names or the categories are all mixed up and disorganized. It would be confusing for anyone to make sense of it, especially for a computer system. When information is labeled consistently and organized in a clear structure, it is much easier for computer systems to work with it and deliver meaningful and tangible results.
Is it Easy to Access?
If your data is scattered across different departments or locked in systems that don’t talk to each other, it becomes hard to use. Ideally, everything should be stored in a shared, central place where it’s easy to find and update the data.
That way, anyone who needs the data, or any tool that uses it, can work with the most current and complete version of the data.
Does the Data Match the Job You Want Done?
Not all data is useful for every problem. If you’re trying to improve how you forecast customer demand, for example, you need data that reflects real buying patterns, such as seasonal shifts, rare trends, and even the oddball purchases.
The data needs to be a good match for the particular set of situations you’re trying to solve.
Why Does AI-Ready Data Matter?
The importance of AI-ready data cannot be overstated. AI models are only as good as the data they are trained on. Poor-quality data can lead to inaccurate predictions, biased decisions, and ultimately, failed AI projects.
For instance, Gartner emphasizes that AI-ready data must be representative of the use case, capturing every pattern, error, and outlier necessary for training or running an AI model effectively.
Moreover, the process of preparing data for AI is often referred to as data preparation, which can account for up to 80% of the total workload in AI initiatives.
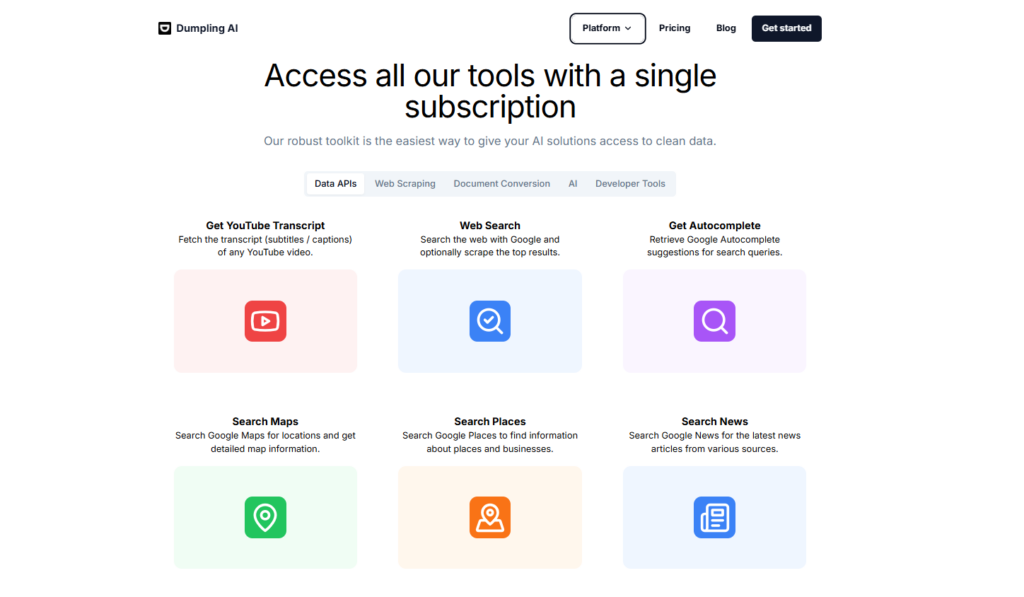
This certainly highlights the significant effort required to ensure that the data is suitable for AI applications, with tools like Dumpling AI, assisting in this process by providing frameworks to assess and enhance data readiness, ensuring that the AI models are built on a solid foundation.
How Can You Prepare Your Data for AI?
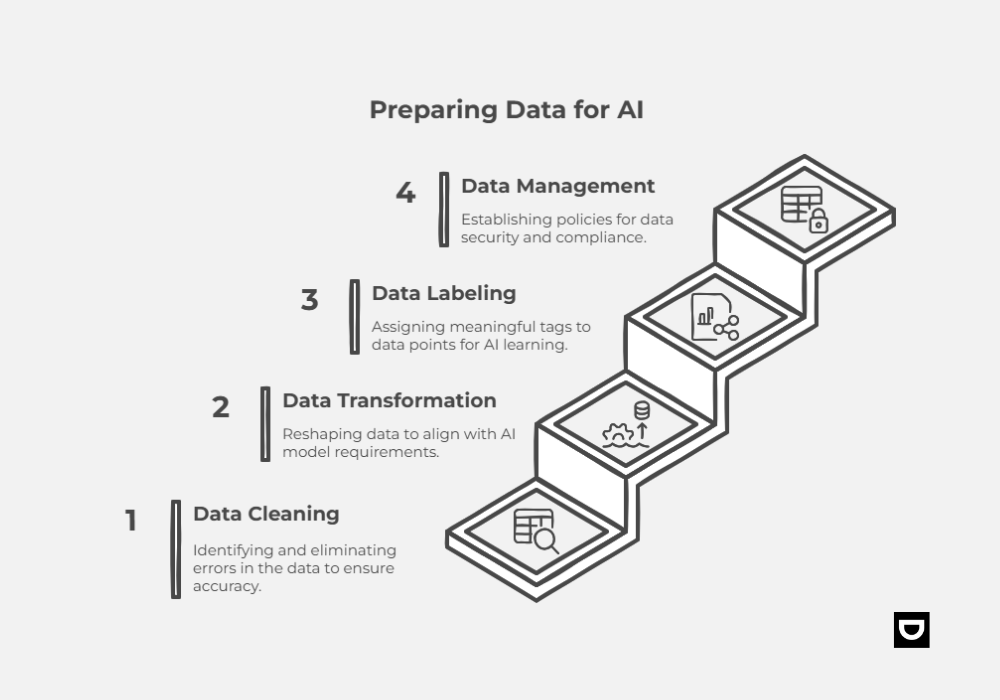
Preparing data for AI involves several critical steps, which include:
Step 1: Data Cleaning
This is a critical process that involves identifying and eliminating errors in the data, such as duplicate data entries and inconsistencies in your dataset, to ensure the accuracy and quality of the information you’re working with. To guarantee data quality, data cleaning is important to eliminate mistakes, duplication, and inconsistencies from your data.
Step 2: Data Transformation
This step involves reshaping or reformatting your data so that it aligns better with the specific input format or structure required by the AI models. This step ensures the adaptation of your data structures to meet the specific requirements of the AI models.
Step 3: Data Labeling
Data labeling is the process of assigning meaningful tags or labels to individual data points, such as images, text, or video frames, so that the AI systems can learn from them. In supervised learning, this stage is essential since labelled data aids the models in producing precise classifications or predictions.
Step 4: Data Management
This is a broader strategy that involves establishing policies and procedures that regulate how the data is managed, accessed, and protected. This step encompasses the oversight of data security, user privacy, and compliance with relevant legal or industry standards.
Metadata Management
This type of management acts as a supporting framework that maintains detailed records about your data, such as its source, structure, meaning, and how it’s used, so that teams can better understand, trace, and utilize it effectively.
This involves maintaining detailed information about your data sources, structures, and usage.
Why Does AI-Ready Data Matter?
When your data is clean, trustworthy, and easy to access, you’re laying the foundation for AI systems that are not only smarter but also faster and more effective in producing real, usable results.
Now, imagine bringing in the AI Data Readiness Inspector: aka AIDRIN. Think of it as a report card for your data’s readiness for AI. As AIDRIN doesn’t just skim the surface. It dives deep into assessing critical dimensions like how complete your dataset is, whether there are outliers or duplicates, the importance of individual features, and any imbalances in your data classes.
It also evaluates fairness and privacy risks. Plus, it ensures your data aligns with the FAIR principles: Findable, Accessible, Interoperable*, and Reusable*.
What Are Real-Life Examples of AI-Ready Data in Action?
Example 1: Deloitte’s Customer Data Strategy.
Deloitte strongly advocates for building a solid, AI-ready data foundation as a critical step for any organization looking to implement generative AI successfully.
They stress that having reliable, well-organized, secure, and easily accessible data isn’t just a technical requirement but a strategic advantage. Without these elements in place, organizations risk basing their AI models on flawed or incomplete information, which could very much undermine your results.
To address this, Deloitte recommends structured practices such as using metadata management techniques and developing comprehensive data dictionaries.
These tools help teams understand where their data comes from, what it means, and how it should be used, paving the way for more consistent, trustworthy insights from AI systems.
Example 2: Oracle’s Approach to AI Integration
Oracle brings a slightly different perspective by focusing on the practical side of embedding AI into its technology ecosystem. They emphasize that for AI tools to be effective, they must be powered by high-quality, well-integrated data. Simply having data isn’t enough; it needs to be clean, consistent, and aligned across systems.
Oracle encourages organizations to take a systematic approach, prioritizing robust data management practices, clear security protocols, and strong privacy protections. These elements are important, not only for operational efficiency but also for building trust in AI-driven outputs.
The Bigger Picture
Together, these Deloitte and Oracle examples express a message: organizations that engage in data preparation for AI through strategic strategy, structure, and protections are more likely to achieve substantial commercial benefits.
AI doesn’t work in a vacuum; it thrives on high-quality data, and managing that data correctly is important to getting the most from your AI investments.
Conclusion
AI-ready data is the foundation for successful AI applications. Ensuring that data is clean, organized, and matched with specified use cases is critical for AI models to function correctly and consistently.
Preparing data for AI requires rigorous activities such as cleaning, converting, labelling, and regulating data. Using tools and frameworks can help to streamline the process, making it more efficient and productive.
By investing in AI-ready data, organizations provide the groundwork for successful AI efforts, allowing them to fully realize the potential of artificial intelligence.
Start Preparing Today
FAQs
What is AI-ready data?
AI-ready data is data that is clean, structured, and aligned with specific AI use cases, enabling AI models to process and analyze it effectively.
Why is AI-ready data important?
AI-ready data ensures that AI models can make accurate and reliable predictions, reducing the risk of biased or incorrect outcomes.
How can I assess if my data is AI-ready?
Assessing AI readiness involves evaluating data quality, structure, completeness, and alignment with specific AI use cases. Tools like AIDRIN can assist in this assessment.
What steps are involved in preparing data for AI?
Preparing data for AI includes cleaning, transforming, labeling, governing, and managing metadata to ensure data is suitable for AI applications.
Can tools like Dumpling AI help in preparing AI-ready data?
Yes, tools like Dumpling AI provide frameworks and assessments to evaluate and enhance data readiness for AI applications.